Getting Started with Decentralized AI
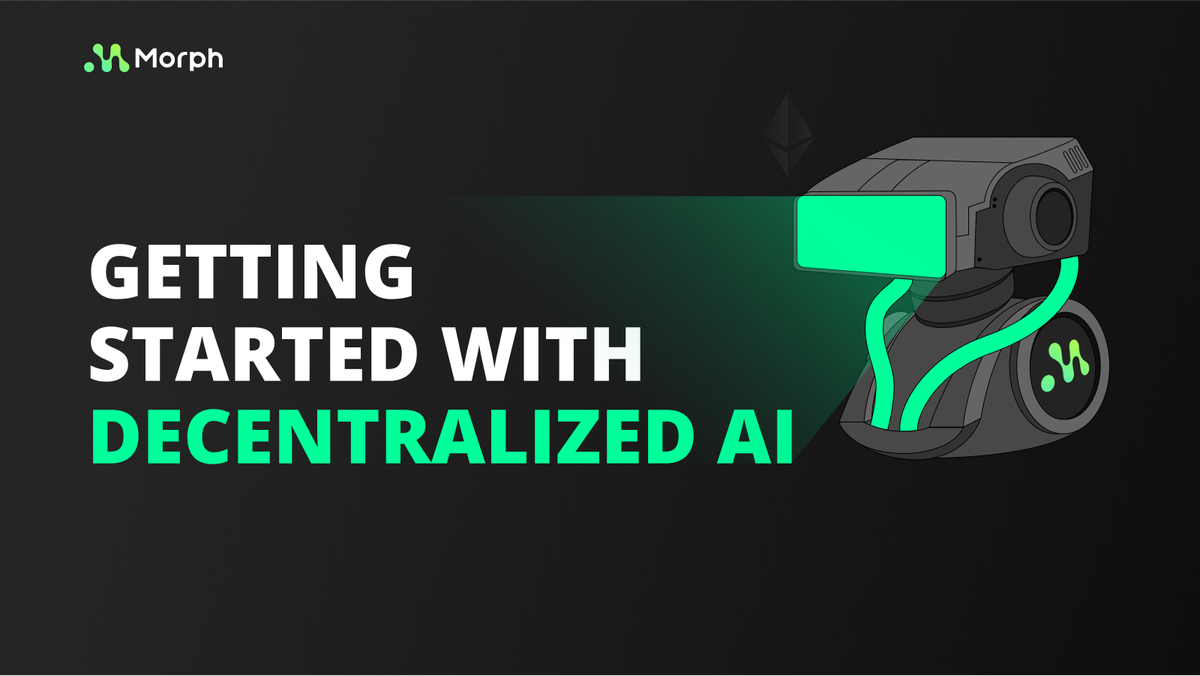
Welcome to the web3 tech series - This newsletter will delve into both our core tech stack and the latest developments in the web3 ecosystem, published every Friday. In our third edition, we'll deep dive into decentralized AI and its importance.
Let’s dive into it.
The Vision of Decentralizing AI
AI and crypto are two transformative technologies that are beginning to converge in powerful ways. Crypto provides AI with a permissionless, trustless, and composable settlement layer, enabling use cases like providing accessible hardware through decentralized compute systems, building AI agents capable of complex tasks requiring value exchange, and developing identity and provenance solutions. Meanwhile, AI enhances the crypto ecosystem in many of the same ways it improves web2 - by providing better user experiences for users and developers via large language models, and significantly improving smart contract functionality and automation. If decentralized AI is successful, there are many benefits:
- Democratize access to AI: Allow individuals and small organizations to access the same opportunities as larger corporations to access and train AI models
- Increase transparency: Democratize how AI models are developed and used, making it easier to understand and trust decisions made by AI
- Encourage innovation: Open up the development of AI to a broader community and promoting self-sovereign ownership over data, AI, and agents
What If We Don't Decentralize?
If AI is structured the same way as companies like Meta and Google, there are several significant risks and issues, including:
- Monopolization of Technology: Centralized entities could limit access to AGI for individuals and organizations,
- Privacy and Security: Centralized data storage is a prime target for breaches, risking personal and sensitive data,
- Lack of Transparency: Centralized AI often operates as black boxes, making it difficult to understand and challenge decisions
If AI remains centralized, the future could see increased inequality in access to technology, greater risks to privacy and security, and continued challenges in ensuring fair and unbiased AI systems.
Use Cases and Examples
- AI Agents / Apps: Agents are trained models that can autonomously execute complex tasks for users. Since blockchains enable agents to hold funds in wallets, they can seamlessly transact and compose with other on-chain services. Examples include: Operator and Myshell AI
- Data Labeling: Facilitating the labeling, provision, and compensation of data for training AI models. For instance: Vana and Get Grass focus on crowdsourcing data labeling and ensuring fair compensation.
- Verified Compute: Leveraging technologies like zero-knowledge proofs to authenticate AI computations. Examples include: Ora Protocol and Modulus Labs, which ensure the integrity and authenticity of AI computations.
- Data Availability and Storage: Solutions for storing vast amounts of AI model data and parameters. Examples: Arweave and Filecoin, providing decentralized storage solutions for AI data.
- Model Training: Platforms that incentivize model creation, decentralized inference, and training. For example: Bit Tensor and Allora Network, which focus on decentralized AI model training and inference.
- Decentralized Compute: optimizing the use of idle GPUs by connecting users and suppliers. Examples include: Akash Network and io.net which provides decentralized computational resources for AI tasks.
Wrapping it Up
Hopefully this guide has provided you the essential information to know more about decentralized AI. We encourage you to provide feedback and ask questions, and subscribe to our newsletter for future articles.
If you're building something exciting in this space, and you're looking to integrate it in an EVM chain - reach out. Morph it's looking for great projects.
You can also join our discord channel or follow us on Twitter.